Which is greater? The number of atoms in the universe or the number of chess moves?
We explore AI's mind blowing processing ability, from winning chess to finding new galaxies.
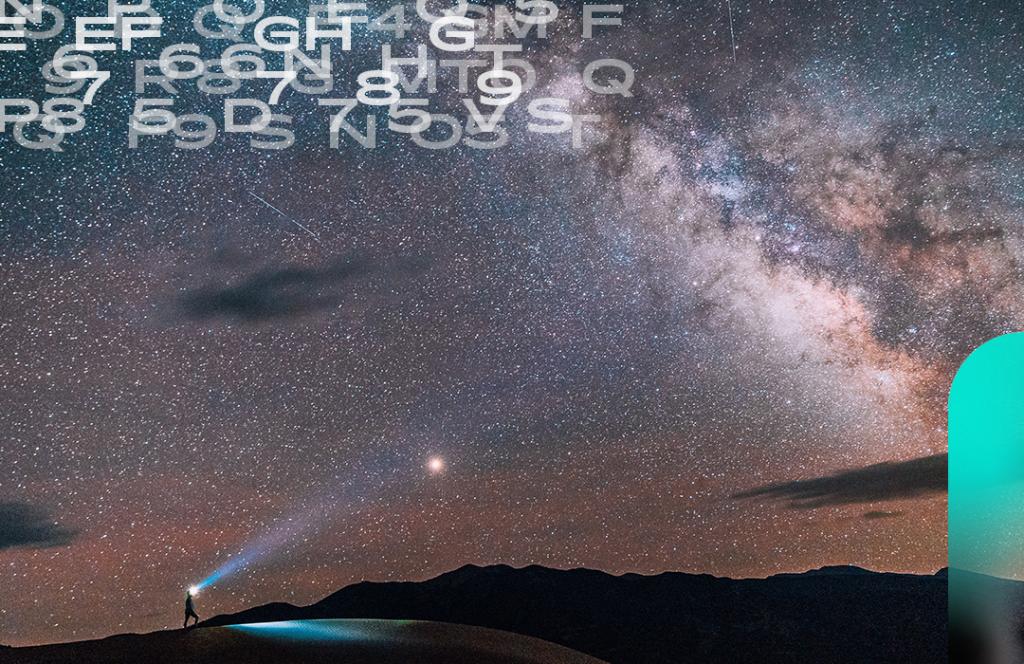
The question came from Claude Shannon, inventor of ‘Information Theory’ in 1948. The theory uses mathematics to understand the rules governing the transmission of messages through communication systems, applicable to everything from computer code, speech and music, to the dancing of bees. Using maths and logic to understand the world around him, it wasn't long before Shannon began to wonder if a computer could beat a human at games, such as chess. In 1950 he wrote a paper asserting this possibility, but it wasn’t until the 1970’s that computers began to defeat humans at the game – generally poor players who made silly mistakes. But they could not defeat Grand-Masters. That did not happen until 1996 when DeepBlue beat Gary Kasparov. The following year the improved DeepBlue beat him 31/2-21/2.
So why did it take so long? Remember the question at the start?
There are between 1078 to 1082 atoms in the observable universe. That’s between ten quadrillion vigintillion and one-hundred thousand quadrillion vigintillion atoms. Which is a lot. But...amazingly, there are even more possible variations of chess games than there are atoms in the observable universe.
This is the Shannon Number and represents all of the possible move variations in the game of chess. It is estimated there are between 10111 and 10123 positions (including illegal moves) in Chess. (If you rule out illegal moves that number drops dramatically to 1040 moves. Which is still a lot!).
"There are even more possible variations of chess games than there are atoms in the observable universe."
You might think, ‘well a computer has conquered the most complicated game in the world there’s nothing left for them to do?’ and you’d be...wrong! There is a game with even more possible moves and variations and it is called Go. Thought to have originated in China over 4.000 years ago it did not become popular until it arrived in Japan around the year 500. It is played extensively in SE Asia: professionals start learning the game as very small children and spend all their lives perfecting their ability.
Go has more than 10170 moves...making it a googol times more complicated and varied than Chess and dwarfing the number of atoms in the Universe!
Do you think a computer or Artificial Intelligence could ever master a game this complicated in your lifetime?
Amazingly, it already has. Enter AlphaGo. In 2015 it played its first match against reigning three-time European Champion Mr. Fan Hui, and beat him 5-0.
In March 2016, the AI then competed against legendary Go player, eighteen-time world title winner Mr. Lee Sedol. It's said that Sedol is to Go what Federer is to tennis, yet, with 200 million people watching world wide, AlphaGo beat him 4-1 in a competition in Seoul, South Korea.
All Go players are ranked; an absolute beginner is ranked as Kyu 30. As they improve the move towards the rank Kyu 1. As they continue to improve they then join the Dan ranks, starting at level 1 and aim for (but rarely reach) level 9 Dan. There are currently just over 100 9 Dan players in the world. AlphaGo is one of them.
There's more...
The company that created AlphaGo – Deepmind – released a newer more powerful version, AlphaGo Zero.
According to Deepmind: “AlphaGo learnt Go by playing thousands of matches with amateur and professional players, AlphaGo Zero learnt by playing against itself, starting from completely random play...and then by playing against the strongest player in the world, AlphaGo.
This powerful technique is no longer constrained by the limits of human knowledge. Instead, the computer program accumulated thousands of years of human knowledge during a period of just a few days. Go Zero quickly surpassed the performance of all previous versions and also discovered new knowledge, developing unconventional strategies and creative new moves, including those which beat the World Go Champions Lee Sedol and Ke Jie...”
Now you may think:
"So what? A machine can play a game, big deal."
The big deal is that it has been able to make new discoveries, novel approaches. That AI can have ‘creative moments’ suggest that AI can be used to enhance human ingenuity rapidly.
When dealing with vast amounts of information, when attempting to understand lots of data (particularly mathematical) the human mind can become overwhelmed and tires quickly. An AI doesn’t have those problems. AlphaGo Zero learnt thousands of years of human knowledge in just a few days. Applying that ability to other areas will allow patterns and discoveries that might otherwise be hidden or take a long time to discover by people alone.
How does an Artificial Intelligence learn?
That’s a really good question and it is both simple and very clever. There are three levels of learning for an AI: Artificial Intelligence, machine learning, and deep learning.
Artificial Intelligence: is the lowest level of computer ‘intelligence.’ It mimics human learning by making decisions based on options and checks them with stored information: Is it round or curvy? Is it green or yellow? Is it a lime or a banana?
Machine Learning: comes from experience. Are all round green things limes? Can they be apples? Is it bigger than a certain size? Therefore, based on what it has ‘learnt’ from option choosing it can say what the object is.
Deep Learning: is a subset of Machine Learning, the software can train itself (using hidden layers called Neural networks) to understand its outputs. This approach uses huge amounts of data as it requires the machine to check with its database (experience) to find things it ‘knows’ already to allow it to identify objects.
"That AI can have ‘creative moments’ suggest that AI can be used to enhance human ingenuity rapidly."
What has this got to do with Astronomy?
Well I’m glad you asked, no really I am.
Even though there are more moves in Chess than atoms in the Universe, the Universe is still very, very big. It is estimated that there are 200-350 billion stars in our galaxy (the Milky Way). Our galaxy is a medium sized galaxy. It is believed there may be over a trillion galaxies in the visible universe and many more that we can’t see.
Think of it this way; next time you go to the seaside, grab a handful of sand, or dig a hole in the sand. How many grains of sand do you think there are in your hand or in the pile you’ve just dug? Thousands? Millions maybe? Now look at the whole beach and try to guess how many grains there are.
It is thought that there are more stars in the universe than grains of sand on every beach on Earth. Most of those stars have at least one planet, often many more, orbiting. So there are even more planets than stars...
Astronomers and Astrophysicists deal with lots and lots of data and as technology improves the amount of data collected increases.
There are many new telescopes and observatories under development and soon to come on-line, as well as the space based James Webb Telescope and the Extremely Large Telescope in Chile there is also coming to Chile in 2021 the Large Synoptic Survey Telescope (LSST) also known as the Vera Rubin telescope.
When it begins operation it will take more than 800 panoramic images each night with a 3.2-billion-pixel camera, recording the entire visible sky twice each week.
Each night it will produce 20 TB of data. The images taken by the LSST Camera are so large that it would take 378 4K ultra-high-definition TV screens to display one of them in full size!
How big is a TerraByte? I hear you ask...
1TB is the same as 681 episodes of The Queen's Gambit (one can dream!).
SO much data, you say? You’re right! It’s far more than we humans can work on. That’s just from one telescope...! There are a whole variety of programs, AI, Machine Learning and Deep Learning systems being used by Astronomers and researchers. Because it is more than a human can cope with, trainable neural networks are needed to help with classifying objects and suggesting to Astronomers those that might be interesting to look at more closely.
The European Southern Observatory has developed Morpheus: a deep-learning framework that incorporates a variety of artificial intelligence technologies developed for applications such as image and speech recognition. To help astronomers, Morpheus will work pixel by pixel through the images looking for galaxies! An Older Morpheus result from 2016, working with Hubble, revealed that here were 10 times more galaxies than previously thought.
"An Older Morpheus result from 2016, working with Hubble, revealed that here were 10 times more galaxies than previously thought."
Researchers at Lancaster University have developed at system called Deep-CEE (Deep Learning for Galaxy Cluster Extraction and Evaluation), a novel deep learning technique to speed up the process of finding galaxy clusters.
First discovered in 1950 by George Abell, galaxy clusters are rare but massive objects. Abell spent years scanning 2000 photographic plates with his eye and a magnifying glass and found 2,712 clusters. Galaxy clusters are important as they will help us understand how dark matter and dark energy have shaped our universe.
Deep-CEE builds on Abell's approach replacing the astronomer with an AI model trained to "look" at colour images and identify galaxy clusters. It is a state-of-the-art model based on neural networks, which are designed to mimic the way a human brain learns to recognise objects by activating specific neurons when visualizing distinctive patterns and colours. The AI was trained by repeatedly showing it examples of known, labelled, objects in images until the algorithm learnt to recognise objects on its own.
Deep-CEE will also be used on the Rubin telescope.
Not yet finished (but with Phase 1 already running) is the Square Kilometre Array (SKA); a series of radio telescopes that span continents and will be the largest ever radio telescope. It’s headquarters are in Jodrell Bank in Cheshire.
The majority of the telescopes will be in South Africa and Australia. It will need 2 super-computers to handle all the data. In South Africa there will be 197 radio dishes and in Australia over 131,000 antennae!
Each year SKA will amass 600 PetaBytes of data (or 1.6 PB per day or ~630 Netflix videos a day). To store this data on an average 500 GB laptop, you would need more than a million of them every year. 500GB is the equivalent of 500 hundred lorries full of paper.
But why does the SKA need such immense computing power?
Scientific image and signal processing for radio astronomy consists of several fundamental steps, all of which must be completed as quickly as possible across thousands of telescopes connected by thousands of miles of fibre optic cable. The computers must be able to make decisions on objects of interest, and remove data which is of no scientific benefit, such as radio interference from things like mobile phones.
What about all the rest?
Then of course there are the telescopes, observatories and satellites that are already working: perhaps one of the most famous is the Hubble Space Telescope.
Hubble transmits about 120 gigabytes of science data every week. That would be roughly 1,097 metres (3,600 feet) of books on a shelf. Hubble has been operational for 30 years and has made over 1.5 million observations.
It’s not just about the data, to get that you need to schedule observations. This can be incredibly complicated – timing, location of object, position of spacecraft, rising and setting times and many other variables have to be looked at. To organise observations and timings Hubble uses SPIKE which uses a very fast neural network-inspired, scheduling algorithm to achieve performance humans can only dream of.
We may not have smart cars or personal robots but advances in Artificial Intelligence are already providing profound benefits and discoveries for us all. AI is not our master, it can only learn based on how we program it and what we determine as important...at least for now!